Multiple convolution neural networks approach for online handwriting recognition






4.95/5 (36 votes)
The research focuses on the presentation of word recognition technique for an online handwriting recognition system which uses multiple component neural networks (MCNN) as the exchangeable parts of the classifier.
- Download source - 2.2 MB
- Download source - 6.8 MB
- Download sample - 159.2 KB
- Download lower_case_letter_v2.zip - 5.6 MB
- Download digit_v2.zip - 3.3 MB
- Download capital_letter_v2.zip - 5.6 MB
Abtract
The research described in this paper focuses on the presentation of word recognition technique for an online handwriting recognition system which uses multiple component neural networks (MCNN) as the exchangeable parts of the classifier. As the most of recent approaches, the system proceeds by segmenting handwriting words into smaller pieces (usually characters) which are recognized separately. The recognition results are then the composition of the individually recognized parts. They are sent to the input of a word recognition module in turn to choose the best one by applying some dictionary search algorithms. The proposed classifier overcomes obstacles and difficulties of traditional ones to big character classes. Furthermore, the proposed classifier also has expandable capacity which can recognize another character classes by adding or changing component networks and built-in dictionaries dynamically.
Introduction
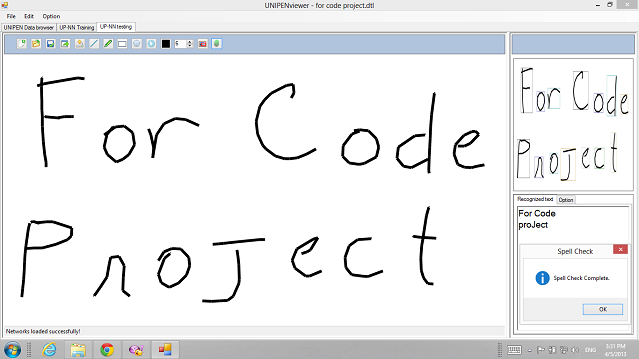
Now a day, touch user interfaces (TUI) are becoming increasingly popular and will play an important role in human-computer interaction. Tablets, smartphones and TUI computers accepting finger or pen based input are becoming an indispensable part of many persons. Using fingers or a pen as an input device takes over many functions of conventional mouse and keyboard. One major advantage of the pen over the mouse is the fact that a pen is a natural writing tool while the mouse is very cumbersome when used as a writing tool. However, it needs a reliable transformation of handwritten text into a coding that can be directly processed by a computer, e.g., ASCII. A traditional transformation model usually includes a preprocessor which extracts each word from image or input screen and divides it into segments. A neural network classifier then finds the likelihoods of each possible character class given the segments. These likelihoods are used as the input to a special algorithm which recognizes the entire word. In recent years, research in handwriting recognition has advanced to a level that makes commercial applications. Nevertheless, significant disadvantages of such single neural network classifiers are complexity in big network organization and expandable capacity.
A high reliable recognition rate neural network can be built easy to recognize a small character class but not to big ones. The larger inputs and outputs make increasing of the neural network’s layers, neurons, connections. Hence, it makes more difficulties to network training process and especially the recognition rate should be significantly decreased. Furthermore, a single neural network classifier only works to a particular character class. It is not exchangeable and or expandable to recognize additional character classes without recreating or retraining the neural network.
This paper presents a new online handwriting recognition system that based on multiple convolutional neural networks (CNNs). Unlike the traditional single neural network classifiers, the new one includes a collection of very high recognition rate component CNNs that work together. Each CNN only recognize correctly to a part of the big character class (digits, alphabet, etc.), but when these networks are combined by programing algorithms they can create a flexible classifier which can recognize differential big character classes by simply adding or removing component CNNs and language dictionaries.
Convolution neural network
Convolutional Neural Networks (CNNs) are a special kind of multi-layer neural networks. Like almost every other neural networks they are trained with a version of the back-propagation algorithm. Where they differ is in the architecture. Convolutional Neural Networks are designed to recognize visual patterns directly from pixel images with minimal preprocessing. They can recognize patterns with extreme variability (such as handwritten characters), and with robustness to distortions and simple geometric transformations.
Fig. 1. A Typical Convolutional Neural Network (LeNET 5)[1]
The convolutional neural network LeNET 5 for handwritten digit recognition has granted reliable recognition rate up to 99% to MNIST dataset. The input layer is of size 32 x32 and receives the gray-level image containing the digit to recognize. The pixel intensities are normalized between −1 and +1. The first hidden layer C1 consists six feature maps each having 25 weights, constituting a 5x5 trainable kernel, and a bias. The values of the feature map are computed by convolving the input layer with respective kernel and applying an activation function to get the results. All values of the feature map are constrained to share the same trainable kernel or the same weights values. Because of the border effects, the feature maps’ size is 28x28, smaller than the input layer.
Each convolution layer is followed by a sub-sampling layer which reduces the dimension of the respective convolution layer’s feature maps by factor two. Hence the sub-sampling maps of the hidden layer S2 are of size 14x14. Similarly, layer C3 has 16 convolution maps of size 10x10 and layer S4 has 16 sub-sampling maps of size 5x5. The functions are implemented exactly as same as the layer C1 and S2 perform. The S4 layer’s feature maps are of size 5x5 which is too small for a third convolution layer. The C1 to S4 layers of this neural network can be viewed as a trainable feature extractor. Then, a trainable classifier is added to the feature extractor, in the form of 3 fully connected layers (a universal classifier).
Fig. 2. A convolution network based on Dr. Partrice Simard’s model
Multiple component neural networks classifiers
Recognition rate of a convolution neural network is really high to small character classes such as digits or English alphabet (26 characters). However, creating a larger neural network that can recognize reliably a bigger collection (62 characters) is still a challenge. Finding an optimized and large enough network becomes more difficult, training network by large input patterns takes much longer time. Convergent speech of the network is slower and especially, the accuracy rate is significant decrease because bigger bad written characters, similar and confusable characters etc.
The proposed solution to the above problems is taking place of a unique complex neural network by multiple smaller networks which have high recognition rate to these own output sets. Each component network has an additional unknown output (unknown character) beside the official output sets (digit, letters…). It means that if the input pattern is not recognized as a character of official outputs it will be understand as an unknown character.
Fig. 3. A MCNNs online handwriting recognition system
Character recognition module of the classifier is a collection of multiple component neural networks which work simultaneously to the input patterns. A handwritten word is pre-processed by segmenting into isolated character visual patterns []. These patterns then are given to the inputs of all component neural networks which will recognize likelihoods of each own character class. A visual pattern can be recognized by one, some or all component networks because there are several similar characters in differential classes. If a network cannot recognize the pattern as a likelihood of its own character class, it will return an unknown character (null character). The module’s output result is a table of possible characters which is composed to possible words such as “Exper1, Expert, ExperJ, EXper1, EXpert, EXperJ” in the above example. Unknown characters (null characters) are not used in word composition. These words then are given to next word recognition module in turn to choose the most corrected one becoming the output of overall classifier. In this example the “Expert” word will be chosen.
Fig. 4. Output of MCNNs classifier module
The algorithm of word composition uses in character recognition module:Global variables:
- charMatrix = List<List<Char>> {{E},{x,X},{p},{e},{r},{1,t,J}}// character table
- words =List<string> //list of composed word.
- startIndex: default is 0
- baseWord: default is “
void GetWords(int startIndex, String baseWord)
{
String newWord = "";
if (startIndex == charMatrix.Count - 1)
{
for (int i = 0; i < charMatrix[startIndex].Count; i++)
{
newWord = String.Format("{0}{1}", baseWord, charMatrix[startIndex][i].ToString());
words.Add(newWord);
}
}
else
{
for (int i = 0; i < charMatrix[startIndex].Count; i++)
{
newWord = String.Format("{0}{1}", baseWord, charMatrix[startIndex][i].ToString());
int newIndex = startIndex + 1;
GetWords(newIndex, newWord);
}
}
}
The word recognition module is in fact a spell checker which uses several dictionary search algorithms and word corrections techniques to get the best meaning word. All possible words from character recognition module are given to the dictionary search sequentially. If one of the words is found in built-in dictionaries it will be the output word of classifier. Otherwise, some word correction techniques will be applied for choosing the most corrected word in automatic mode or showing a list of similar words to user in manual mode. Some of these techniques are:
- swap out each char one by one and try all the chars in its place to see if that makes a good word.
private bool ReplaceChars(String word, out String result)
{
result = "";
bool isFoundWord = false;
foreach (WordDictionary dictionary in Dictionaries)
{
ArrayList replacementChars = dictionary.ReplaceCharacters;
for (int i = 0; i < replacementChars.Count; i++)
{
int split = ((string)replacementChars[i]).IndexOf(' ');
string key = ((string)replacementChars[i]).Substring(0, split);
string replacement = ((string)replacementChars[i]).Substring(split + 1);
int pos = word.IndexOf(key);
while (pos > -1)
{
string tempWord = word.Substring(0, pos);
tempWord += replacement;
tempWord += word.Substring(pos + key.Length);
if (this.TestWord(tempWord))
{
result = tempWord.ToString();
isFoundWord = true;
return isFoundWord;
}
pos = word.IndexOf(key, pos + 1);
}
}
}
return isFoundWord;
}
- try swapping adjacent chars one by one.
private bool SwapChar(String word, out String result)
{
result = "";
bool isFoundWord = false;
foreach (WordDictionary dictionary in Dictionaries)
{
for (int i = 0; i < word.Length - 1; i++)
{
StringBuilder tempWord = new StringBuilder(word);
char swap = tempWord[i];
tempWord[i] = tempWord[i + 1];
tempWord[i + 1] = swap;
if (this.TestWord(tempWord.ToString()))
{
result = tempWord.ToString();
isFoundWord = true;
return isFoundWord;
}
}
}
return isFoundWord;
}
- try omitting one char of word at a time.
- try inserting a new character before every letter.
private bool ForgotChar(String word, out String result)
{
result = "";
bool isFoundWord = false;
foreach (WordDictionary dictionary in Dictionaries)
{
char[] tryme = dictionary.TryCharacters.ToCharArray();
for (int i = 0; i <= word.Length; i++)
{
for (int x = 0; x < tryme.Length; x++)
{
StringBuilder tempWord = new StringBuilder(word);
tempWord.Insert(i, tryme[x]);
if (this.TestWord(tempWord.ToString()))
{
result = tempWord.ToString();
isFoundWord = true;
return isFoundWord;
}
}
}
}
return isFoundWord;
}
- split the string into two pieces after every char. If both pieces are good words make them a suggestion etc.
private bool TwoWords(String word, out String result)
{
result = "";
bool isFoundWord = false;
for (int i = 1; i < word.Length - 1; i++)
{
string firstWord = word.Substring(0, i);
string secondWord = word.Substring(i);
if (this.TestWord(firstWord) && this.TestWord(secondWord))
{
string tempWord = firstWord + " " + secondWord;
result = tempWord;
isFoundWord = true;
return isFoundWord;
}
}
return isFoundWord;
}
By using multiple differential language dictionaries simultaneously in the spell checker, the proposed classifier can recognize correctly differential languages if there are component neural networks that can recognize these languages’ character classes.
public NNTestingControl()
{
InitializeComponent();
bitmap = null;
networks = null;
textSpellControl1.SpellChecker = this.multipleSpelling;
//English dicionary
multipleSpelling.Dictionaries.Add(this.wordDictionary1);
//France dictionary
//multipleSpelling.Dictionaries.Add(this.wordDictionary2);
//Italian dictionary
//multipleSpelling.Dictionaries.Add(this.wordDictionary3);
}
Experiments and results
The demo uses three component CNNs to recognize 62 English characters class. It can get high recognition rate to my own word drawing samples. I do hope this project can help anyone want to study on handwrting recognition. At present I do not have time to continue it, but I hope someone will develop it to a good opensoure project. This is the full sourcecode of all my previous articles. All information of this project can be found here.
History
01/04/2013: update some pictures