In this article, we discuss the nature and importance of time series data.
Introduction
We all know how trendy AI is nowadays. There is a lot of documentation and articles about the most common AI applications: image classification, object detection, regression, and so on. If you see something about data sequences, it’s likely to be about text data for topic classification or something along these lines.
What about anomaly detection in sequence data? This definitely requires attention, especially for the data that comes in real time, such as time-series weather measurements coming from sensors, stock or cryptocurrency prices, or even sensors installed in a factory. Just imagine being able to detect future anomalies in a ship engine – so that you can shut it down before it collapses – wouldn’t that be amazing?
This series of articles will guide you through the steps necessary to develop a fully functional time series forecaster and anomaly detector application with AI. Our forecaster/detector will deal with the cryptocurrency data, specifically with Bitcoin. However, after following along with this series, you’ll be able to apply the concepts and approaches you’ve learned to any data type of similar nature.
To fully benefit from this series, you should have some Python, Machine Learning, and Keras skills. The entire project is available in my GitHub repository. You can also check out the fully interactive notebooks here and here.
Understanding Time Series Data in the AI Context
Let’s start with a brief explanation of time-series. If you’re familiar only with the traditional Machine Learning classification and regression problems, time series data would come as a bit of a surprise. It is a completely different kind of modeling task that can take some time to get familiar with. Its temporal structure makes the observations to have an order that cannot be treated like in any other type of analysis.
Time series data could be described as sequences of observations equally spaced in time. This type of data can be found literally everywhere. You can find it in the weather info as a meteorologist, in the stock/cryptocurrency prices as an economist or trader, in an electrocardiogram if you’re in the health field, in seismological readings – and in data coming from any kind of sensor. Imagine what you could achieve by analyzing it, especially in the AI context.
Let’s look at an example. The table below shows the first 5 rows of this dataset, which contains the weather readings captured by sensors in New York. Keep an eye on its index and notice that they are equally spaced in time – one record in one day:
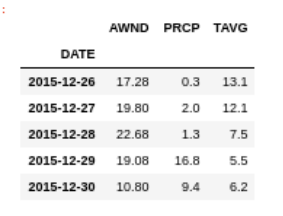
Each column represents a variable that describes a phenomenon sampled every day. AWND corresponds to average wind speed in kilometers per hour, PRCP – to average precipitation in millimeters, and TAVG – to average temperature measured in Centigrade.
Let’s display all the available TAVG data in a scatter plot:

As you can see, keeping the sequence of the data points is crucial to understand its underlying structure. In this case, it represents an obvious trend, an aspect that you wouldn’t notice if you didn’t respect the sequential order.
The above concept provides a basis to several tasks, especially in the data science and Machine Learning fields. Just imagine being able to forecast tomorrow’s weather (forecasting is the task of predicting a future value given a series of past ones). Whether it’s going to rain or, in more advanced cases, whether tomorrow’s temperature represents an anomaly in the weather history (anomaly detection).
This idea can be extrapolated to any data of similar data type. For example, you could predict tomorrow’s Bitcoin price. Will it represent an anomaly in its market? And if yes, how about making a decision to buy or sell based on it… wouldn’t that be exciting?
Next Step
In the next article, we’ll discuss pre-processing of the time series data for forecasting and anomaly detection tasks based on Bitcoin’s historical price. Stay tuned!
History
- 24th February, 2021: Initial version